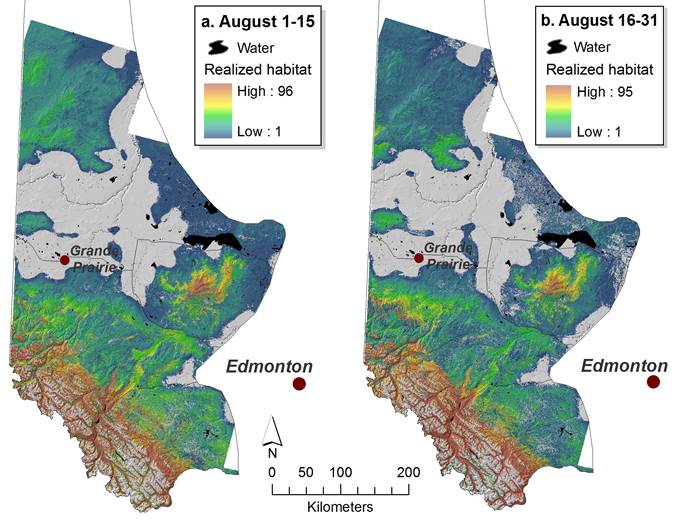
Dynamic Regeneration of Grizzly Bear Food Model
This is a GIS tool developed by the fRI Research Grizzly Bear Program that regenerates the spatial grizzly bear food models developed by Dr. Scott Nielsen.
The value of a spatial habitat model to landscape planners lies in its ability to predict the spatial distribution of some habitat attribute—in this case, grizzly bear food—in areas where actual empirical data is lacking.
To model grizzly bear habitat quality, the fRI Research Grizzly Bear Program (GBP) has used a Resource Selection Function (RSF) modeling approach that depicts the relative probability of occurrence of grizzly bears over their entire range in western Alberta, an area of more than 250,000 km2. RSF models are based on the premise that animals do not use habitat types according to their availability, but preferentially select certain habitat types over others. To develop an RSF, GPS bear locations are overlaid with spatial layers representing some particular habitat attribute, and the distribution of locations relative to the attribute is compared with a set of random locations. These models are high-resolution (30 meter x 30 meter pixel size), seasonally specific, and independently validated with GPS data and DNA-based population inventory survey results.
Developing a reliable RSF, however, requires abundant GPS data for model-building and validation. To complement the RSF model where GPS data was sparse, the GBP used a food-based modeling approach, and developed probability of occurrence models for 20 key grizzly bear food species groups. The importance of each food species was weighted according to scat analysis for each of 10 semimonthly periods over the growing season. Each of the 20 food species models, and the 10 seasonal composite food models, is a continuous variable represented spatially by a raster GIS layer, with 30-meter horizontal resolution (cell size). Each layer is calculated on a pixel-by-pixel basis by a MapAlgebra expression in ESRI’s Spatial Analyst Raster Calculator.
For food-limited populations, nutritional-landscape modeling offers an alternative approach to habitat modeling that defines habitat in units that relate the nutritional needs of individual animals and seasonal patterns of availability. Food-based habitat modeling thus has several advantages over RSF modeling, in that habitat quality is directly linked to resources that influence reproduction; high-use areas associated with activities like bedding and movement are not emphasized as critical habitat; the temporal resolution is bimonthly rather than three seasons per year; and model development does not require numerous GPS locations in the area of interest.
While RSF and food-based habitat models are valuable tools for decision support in conservation planning, they are limited by being static layers. The challenge for land and resource planners in Alberta is that the landscape in grizzly bear range is being continually altered, both by natural processes such as fire, infestation, and natural forest succession, as well as by human activities such as logging, mining, farming, settlement, recreation, and oil and gas extraction. Superimposed on these changes is the prospect of rapid climatic shifts due to rising concentrations of atmospheric greenhouse gases. Static habitat models quickly become out of date.
A means to dynamically update the habitat models enables planners to not only keep their habitat maps current, but also to forecast the outcomes of various development scenarios and compare them quantitatively. The habitat models thus become not only spatially predictive, but temporally predictive as well. Just as a reliable forecast of tomorrow’s weather is far more valuable than a report on yesterday’s weather, the ability to predict changes in habitat quality is of great benefit to land and resource managers.
To meet this objective, the GBP developed simple GIS applications, written in the Python language and integrated with ESRI ArcGIS Desktop, that rapidly regenerate the RSF and food models for a user-specified area of interest. The user has the option to input a shapefile or geodatabase feature class representing a new disturbance event, such as forest harvest cut block, a new well site, or new road. This feature will be incorporated into the input layers, and the regenerated models will reflect this new landscape condition.
The ability to quickly generate multiple habitat forecasts for different change scenarios allows planners to anticipate the outcomes of planned activities and compare the costs and benefits of various options. The modeled landscape upon which these short-term plans are imposed can itself be altered to reflect long-term changes in landscape composition resulting from the impacts of climate change, wildfire patterns, and forest harvest regimes, and these outcomes can be used for prioritizing areas for population recovery.
Ultimately, food models will be linked to energy budgets for individual animals, and this data will be used to determine a landscape unit’s carrying capacity, which in turn can be used to set targets for population recovery.
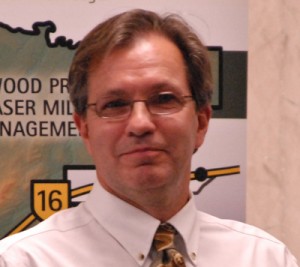
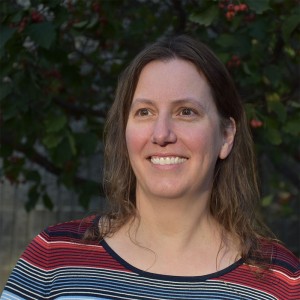