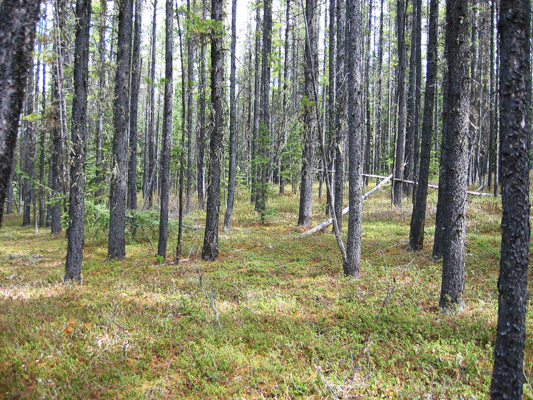
Abstract
Food and habitat resources are critical components of wildlife management and conservation efforts. The grizzly bear (Ursus arctos) has diverse diets and habitat requirements particularly for understory plant species, which are impacted by human developments and forest management activities. We use light detection and ranging (LiDAR) data to predict the occurrence of 14 understory plant species relevant to bear forage and compare our predictions with more conventional climate- and land cover-based models. We use boosted regression trees to model each of the 14 understory species across 4435 km2 using occurrence (presence–absence) data from 1941 field plots. Three sets of models were fitted: climate only, climate and basic land and forest covers from Landsat 30-m imagery, and a climate- and LiDAR-derived model describing both the terrain and forest canopy. Resulting model accuracies varied widely among species. Overall, 8 of 14 species models were improved by including the LiDAR-derived variables. For climate-only models, mean annual precipitation and frost-free periods were the most important variables. With inclusion of LiDAR-derived attributes, depth-to-water table, terrain-intercepted annual radiation, and elevation were most often selected. This suggests that fine-scale terrain conditions affect the distribution of the studied species more than canopy conditions.
For access to the full article, go here.
Citation
Nijland, W., Nielsen, S. E., Coops, N. C., Wulder, M. A., & Stenhouse, G. B. (2014). Fine-spatial scale predictions of understory species using climate- and LiDAR-derived terrain and canopy metrics. Journal of Applied Remote Sensing, 8(1), 083572. doi:10.1117/1.JRS.8.083572