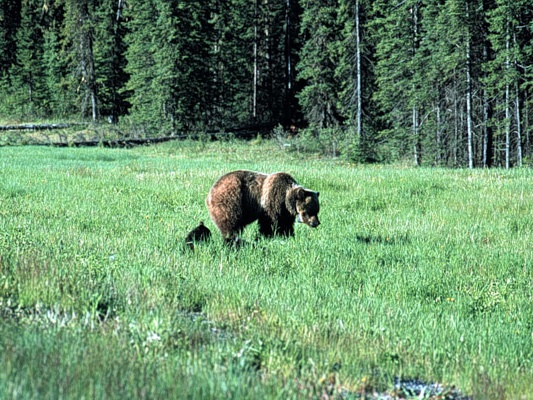
Abstract
There is a vital need to understand wildlife movement and space-use patterns to inform conservation efforts given current rates of anthropgenic environmental change. Grizzly bears (Ursus arctos), among other large predators, are especially vulnerable to landscape change given their need to travel across large areas in search of seasonally available foods. Understand how grizzly bears make movement decisions based on learned knowledge and how these decisions lead to home range size is key in developing effective conservation and management decisions. While previous research has provided insight into bear relationships to various landscape features, many conventional approaches are challenged by the need to understand how complex movement decisions over time allow bears to access habitat providing necessary resources and establish a home range. We present a novel agent-based model (ABM) that simulates individual bear movement decisions at multiple scales that are governed by learning and memory in a dynamic landscape. Using GPS-radio collared data of bear movement in west-central Alberta, our model successfully identifies movement behavours that lead to the emergence of home ranges and provides new insight in to how bears use previously acquired landscape data to maximize use of high-quality areas within a heterogeneous landscape. Future modelling efforts should continue to explore the intricacies of movement behaviour in wide ranging species, including the movement memory interface.
Read the full paper here.
Citation
Zubiria Perez, A., Bone, C., Stenhouse, G. (2021). Simulating multi-scale movement decision-making and learning in a large carnivore using agent-based modelling. Ecological Modelling 452, 109568. doi:10.1016/j.ecolmodel.2021.109568